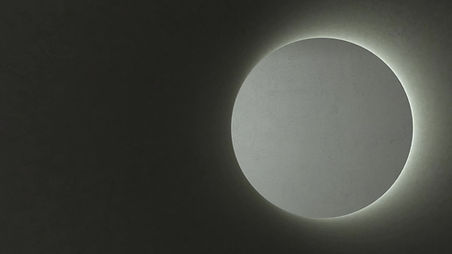
Green A.I. and the Efficiency Lie
24-09-04
Sufficiency Is the Secret to True Green Computing

In the race to develop environmentally sustainable artificial intelligence, much of the focus has been on improving efficiency. However, as we grapple with the growing energy demands of AI systems, it's becoming clear that efficiency alone is not enough. We need to shift our focus to sufficiency.
But what is sufficiency in the context of AI? Sufficiency refers to the principle of using only what is needed to achieve the desired outcome, rather than constantly pushing for more. It's about asking not just "How can we do this more efficiently?" but "Do we need to do this at all?" and "What is the minimum required to accomplish our goal?"
The Limitations of Efficiency
Efficiency gains in AI have been impressive. We've seen the development of more energy-efficient hardware, optimized algorithms, and improved data center management. However, these improvements often fall victim to the rebound effect: as AI becomes more efficient, we use it more, potentially negating the environmental benefits.
For instance, while individual AI models may be more efficient, the trend towards larger, more complex models means overall energy consumption continues to rise. The GPT-3 model, despite being more efficient per parameter than its predecessors, still has a substantial carbon footprint due to its sheer size.
What Embracing sufficiency in AI development means
Right-sizing models: Instead of always opting for the largest, most powerful model, we should choose the smallest model that adequately solves the problem at hand.
Questioning necessity: Before developing or deploying an AI solution, we should critically evaluate whether AI is truly necessary for the task.
Optimizing for constraints: Rather than pushing for ever-increasing accuracy at the cost of computational resources, we should set energy or carbon budgets and optimize within those constraints.
Promoting "small data" approaches: We should explore techniques that can achieve good results with smaller datasets, reducing the energy needed for data storage and processing.
Extending hardware lifecycles: Instead of always upgrading to the latest hardware, we should maximize the use of existing infrastructure where possible.
Achieving sufficiency in AI requires changes at multiple levels
Research: We need more studies on the environmental impact of AI and how to measure and optimize for sufficiency.
Industry: Companies should adopt sufficiency metrics alongside efficiency and performance metrics when developing and deploying AI systems.
Policy: Regulators could introduce sufficiency requirements for AI systems, particularly for public sector applications.
Education: AI curricula should include training on environmental impacts and sufficiency principles.
It's clear that sufficiency must play a central role if we're to achieve truly Green AI. By embracing sufficiency, we can ensure that AI not only becomes more environmentally sustainable but also more focused, purposeful, and aligned with genuine human needs. The future of Green AI lies not in doing more with less, but in doing enough with just what's necessary.
Latest News