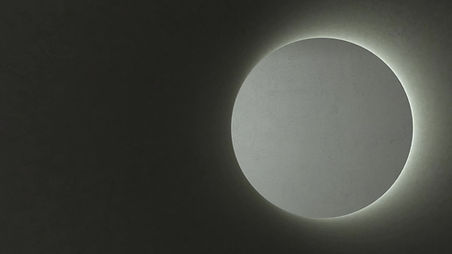
AI Predicting User Behavior
24-03-11
70-85 % correct

Artificial Intelligence has revolutionized many sectors, and the advertising industry is no exception. One of the most intriguing applications of AI in this field is its ability to predict user behavior. But how accurate are these predictions?
AI uses machine learning algorithms to analyze data and make predictions about future outcomes. In the context of advertising, AI systems can be trained to analyze user behavior data, such as browsing history, purchase history, and social media interactions.
These systems can then use this analysis to predict future user behavior, such as what products a user might be interested in or when they might be likely to make a purchase.
The Accuracy of AI Predictions
The accuracy of AI predictions can vary widely depending on several factors,including the quality of the data being analyzed, the sophistication of the AI system, and the complexity of the behavior being predicted.
That said, several studies have shown that AI can be remarkably accurate in predicting user behavior. For example, a study by McKinsey found that AI systems can increase the accuracy of predictive marketing models by up to 20%.
Back to this article's title; what about the 70-85% accuracy results? Well, that is ChatGPT 4 answer. If you ask Google Gemini Pro, it will answer 60-75% results. If you ask them how they calculated that range, here are the variables and parameters they use:
Variables:
Model complexity: The sophistication of the AI model, including the algorithms used and the number of parameters it can learn.
Data quality and quantity: The amount and quality of data used to train the model, including the representativeness and diversity of the data.
User variability: The inherent unpredictability of human behavior and the ability of the model to account for individual differences and context-specific factors.
Parameters:
Accuracy metrics: The specific metrics used to evaluate the model's performance, such as mean absolute error or root mean squared error.
Data splitting: The method used to divide the data into training, validation, and test sets to ensure unbiased evaluation.
Hyperparameter tuning: The process of optimizing the model's hyperparameters, such as learning rate and regularization, to improve performance.
Factors Influencing Accuracy
Several factors can influence the accuracy of AI predictions:
Quality of Data:
AI algorithms are only as good as the data they're trained on. High-quality, diverse, and representative data sets can significantly improve prediction accuracy. Conversely, poor quality or biased data can lead to inaccurate or skewed results.
Algorithm Sophistication:
Not all AI is created equal. The sophistication of the machine learning algorithm used plays a significant role in prediction accuracy. More advanced models like deep learning neural networks can often provide more accurate predictions than simpler models, provided they have enough data to learn from.
Feature Selection:
This refers to the variables or attributes used by the model to make predictions. Effective feature selection can greatly enhance the accuracy of AI predictions. Irrelevant or redundant features can confuse the model and reduce accuracy.
Strategies for Improving AI Prediction Accuracy
Ensemble Methods:
Ensemble methods involve combining the predictions of multiple models to achieve higher accuracy. Techniques like bagging, boosting, and stacking fall under this category. While much of this process can be automated, human oversight is often necessary to choose appropriate base models and ensemble techniques based on the problem at hand.
Bagging: This technique involves creating multiple subsets of the original data and training a model on each subset. The final prediction is typically the average (for regression problems) or majority vote (for classification problems) of the individual model predictions.
Boosting: Boosting trains models sequentially, with each new model attempting to correct the errors made by the previous ones. The final prediction is a weighted sum of the individual model predictions.
Stacking: Stacking involves training multiple different models and then combining their predictions using another model (the meta-model).
Transfer Learning:
Transfer learning involves applying knowledge learned from one task to another related task. This approach can improve accuracy when there's limited data available for the task at hand. Automation can handle much of the transfer learning process, but human expertise is required to identify tasks that are sufficiently related for transfer learning to be effective.
Active Learning:
Active learning is an approach where the AI system actively selects the most informative examples to learn from. This strategy can lead to improved accuracy with less data. While the selection process can be automated to a large extent, human involvement is often necessary to validate the selected examples and provide labels if needed.
Human Intervention in Improving Accuracy
While automation plays a significant role in implementing these strategies, human expertise is still crucial. Humans are needed to:
Choose appropriate models and techniques based on the problem context.
Validate and label data, especially in active learning scenarios.
Monitor and interpret model performance metrics.
Address issues such as bias and fairness that may arise in the model's predictions.
It's important to acknowledge the impressive strides that have been made. Even with conservative estimates placing accuracy around 60-75, and more optimistic ones reaching up to 85, these figures represent a significant achievement.
AI technology has come a long way, evolving from simple rule-based systems to complex models capable of learning intricate patterns and making predictions about future behavior. These advancements are transforming industries, from marketing and advertising to healthcare and beyond, enabling us to understand and serve users in ways that were previously unimaginable.
However, as with any technology, it's essential to remember that AI is a tool. Its effectiveness depends on how well it's used - the quality of the data it's trained on, the appropriateness of the model for the task at hand, and the care taken to avoid bias and ensure fairness.
While there's still room for improvement and challenges to overcome, the progress made so far is undeniably impressive. With ongoing research and development, the predictive capabilities of AI are set to become even more accurate and impactful in the years to come.
Latest News